- My favorite bone conduction headphones have a unique feature - and it's 41% off for Black Friday
- Best Black Friday TV deals 2024: 85 expert-selected deals on QLED, OLED, & more
- Malicious PyPI Package Exposes Crypto Wallets to Infostealer Code
- The special edition Dyson Airwrap bundle is now $499 at Amazon for Black Friday
- The 8TB T5 Evo SSD is almost 50% off at Samsung for Black Friday
The timeline for quantum computing is getting shorter
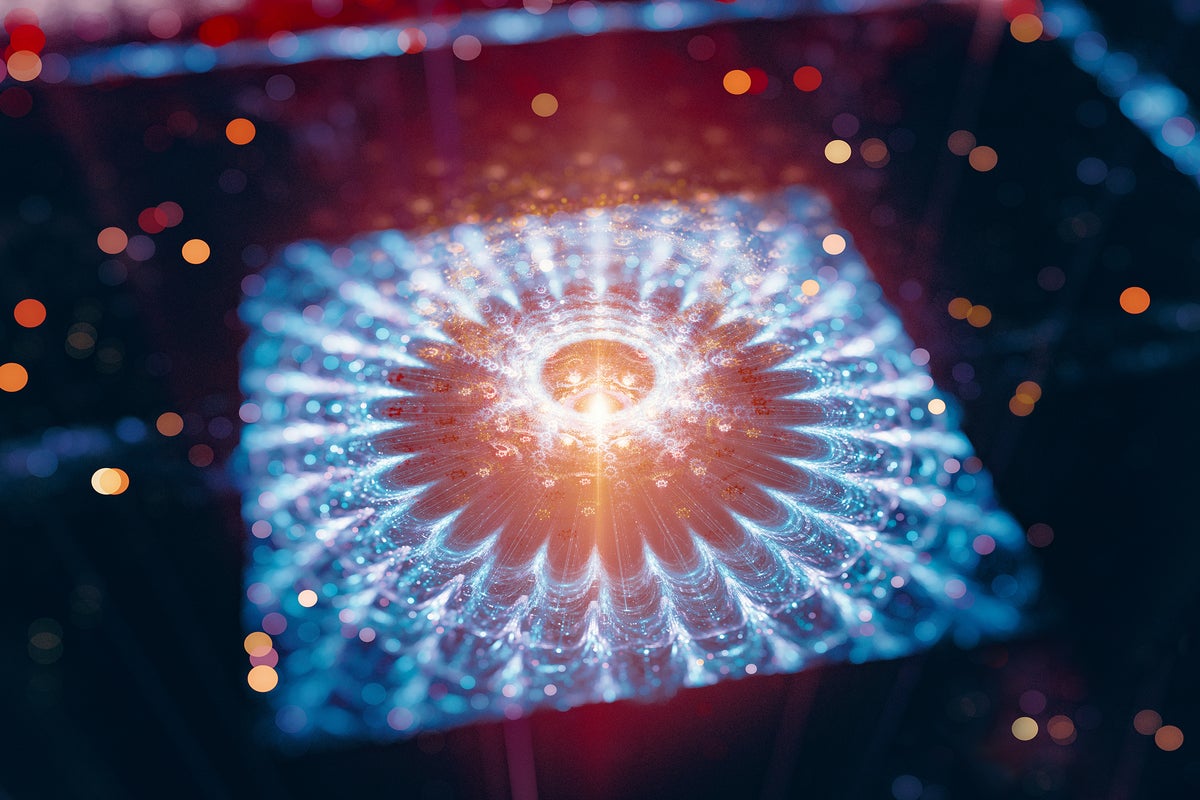
Financial traders rely heavily on computer financial simulations for making buying and selling decisions. Specifically, “Monte Carlo” simulations are used to assess risk and simulate prices for a wide range of financial instruments. These simulations also can be used in corporate finance and for portfolio management.
But in a digital world where other industries routinely leverage real-time data, financial traders are working with the digital equivalent of the Pony Express. That’s because Monte Carlo simulations involve such an insanely large number of complex calculations that they consume more time and computational resources than a 14-team, two-quarterback online fantasy football league with Superflex position.
Consequently, financial calculations using Monte Carlo methods typically are made once a day. While that might be fine in the relatively tranquil bond market, traders trying to navigate more volatile markets are at a disadvantage because they must rely on old data. If only there were a way to accelerate Monte Carlo simulations for the benefit of our lamentably ladened financial traders!
Soon there will be, according to financial services giant Goldman Sachs and QC Ware, a quantum-as-a-service provider that develops applications to run on near-term quantum-computing hardware. Researchers for the two partners reportedly have designed new quantum algorithms for running Monte Carlo simulations on near-term quantum hardware expected to be available in five to 10 years.
There’s a lot to unpack there. First, what is near-term quantum computing hardware? Basically, it’s a flawed and error-prone version of the fully realized version of quantum computing, highly susceptible to environmental “noise” that contaminates results. In practical terms, that means near-term quantum devices have high error rates and will begin returning incorrect results after only a few calculation steps.
I know, sign me up, right? Fortunately, there are quantum algorithms capable of reducing errors while enabling quantum computers to perform Monte Carlo simulations 1,000 times faster than classical methods. Unfortunately, the error-corrected quantum hardware required for these algorithms to run simulations at that speed is 10 to 20 years away.
Goldman Sachs and QC Ware researchers set about trying to find some middle ground between speed of implementation and optimum quantum computing performance.
“By successfully sacrificing some of the speed-up from 1000x to 100x, the team was able to produce Shallow Monte Carlo algorithms that can run on near-term quantum computers expected to be available in five to 10 years,” the two companies said in a press release.
So while there’s no immediate help, financial traders can take comfort in knowing the timeline for faster Monte Carlo simulations has been cut in half. A few short years from now, financial Monte Carlo simulations and 14-team, two-quarterback online fantasy football league with Superflex position shall scarcely look the same. That goes for other endeavors for which quantum computing is expected to be transformative, including healthcare, artificial intelligence, logistics, manufacturing, and national security.
The ability of quantum algorithms to exponentially increase computing speeds will allow enterprises to innovate faster, respond more quickly to market disruptions, and operate more efficiently. That adds up to quite a competitive advantage. CIOs ignore quantum computing at their own peril.
Bonus quantum-computing breakthrough news
Meanwhile, proving there is more than one way to skin a qubit, Los Alamos National Laboratory reports it is using machine learning to develop algorithms that make today’s quantum computers less vulnerable to noise.
In a new paper, the scientific research agency demonstrates how a method called “noise-aware circuit learning” can reduce error rates by two or three times.
Patrick Coles, a quantum physicist in at Los Alamos National Laboratory and lead author on the paper, said the machine learning approach is similar to a person receiving a vaccine that trains the immune system to resist a virus. Machine learning allows quantum algorithms, or circuits, to build resistance to a specific quantum machine’s noise processes. There’s an analogy we all can relate to these days.
Copyright © 2021 IDG Communications, Inc.